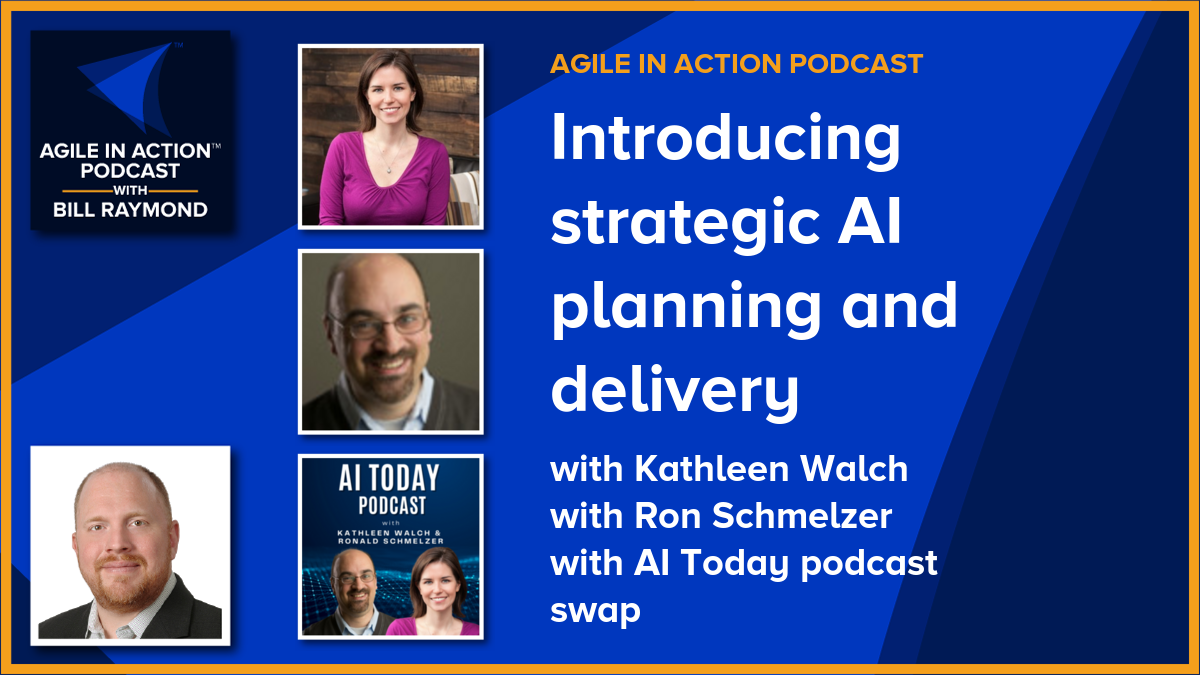
Listen now
Introducing strategic AI planning and delivery
Kathleen Walch, AI Today podcast host and Managing Partner at Cognolytica
Ron Schmelzer, AI Today podcast host and Managing Partner at Cognilytica
AI Today podcast swap, Bill Raymond, founder and host of the Agile in Action with Bill Raymond podcast
- Cognilytica / AI & Data Today
- AI Today podcast website
- AI Today podcast on Apple Podcasts
- AI Today podcast on Spotify
- AI Today podcast on Google Podcasts
About this podcast episode
🔁 Podcast Swap!
More than 70% of AI projects fail. Learn how not to be a statistic ⤵️
Kathleen Walch and Ron Schmelzer, hosts of Cognilytica’s AI Today podcast, share how you can deliver successful artificial intelligence projects.
Additionally, Bill Raymond will appear on the AI Today podcast, sharing his thoughts on using agile techniques to deliver these complex solutions.
In today’s Agile in Action podcast, you will learn the following:
✅ The seven patterns of AI
✅ Why AI projects typically fail
✅ How to successfully deliver AI projects
✅ The importance of data roles on AI teams
✅ Six iterations for creating an AI solution
How the video was created
The script for this video was created using OpenAI’s ChatGPT service.
Here was the ChatGPT prompt:
“I am the podcast host for the Agile in Action with Bill Raymond podcast. My podcast is focused on helping teams and organizations improve the value they deliver to customers using agile techniques. Tomorrow, I am going to interview a team of people from the AI Today podcast. That AI Today podcast teaches their listeners on techniques that help deliver articial intelligence projects. Write the text for a trailer that introduces both podcasts in a positive light in the style of the 1980’s and 1990’s movies that start with “In a World” as if it was Don LaFontaine speaking.”
Here is the resulting script:
In a world where technology advances at lightning speed, two powerful podcasts join forces to bring you the ultimate guide to success.
From the Agile in Action with Bill Raymond podcast, learn how to improve the value your team delivers to customers using cutting-edge agile techniques.
And from the AI Today podcast, discover the secrets to delivering artificial intelligence projects on time and on budget.
Together, the Agile in Action and AI Today podcasts will take you on a journey to the forefront of innovation and help you stay ahead of the competition.
Don’t miss this dynamic duo, available now on your favorite podcast app.
Putting the video together
The resultant script was pasted into Descript, which (among many other things) can generate voice audio from text. Further, Descript generated the audio animation and the transcription. Descript also offers the use of royalty-free videos, which I added.
The whole process took 15 minutes to complete.
Transcript
(transcripts are auto-generated, so please excuse the brevity) Bill Raymond: Hi, this is Bill Raymond. Today, I’m excited to have some podcasts guests here that talk about artificial intelligence or AI.They will be talking about how to successfully deliver AI projects, but I will also be on their podcast to share some of the ideas that I have for successfully managing AI projects. So I figured why not start this podcast with AI generated content? So here we go.
AI Generated Content: In a world where technology advances at lightning speed, two powerful podcasts join forces to bring you the ultimate guide to success. From the Agile in Action with Bill Raymond Podcast learn how to improve the value your team delivers to customers using cutting edge agile techniques. And from the AI Today Podcast discover the secrets to delivering artificial intelligence projects on time and on budget.
AI Generated Content: Together the Agile in Action and AI Today Podcast will take you on a journey to the forefront of innovation and help you stay ahead of the competition. Don’t miss this dynamic duo available now on your favorite podcast app.
Bill Raymond: If you were interested in how I created this intro, go ahead and read the story on the agileinaction.com website. Where you find the AI today podcast interview that you’re about to listen to right now. With that let’s get started. Welcome to the Agile Inaction Podcast with Bill Raymond. Bill will explore how business disruptors are adopting agile techniques to gain a competitive advantage in this fast-paced technology driven market. Hi, and welcome to the podcast today. I’m joined by Kathleen Walch and Ron Schmelzer podcast host for the AI today podcast. And they’re also managing partners at Cognilytica. Hi, Kathleen. Hi, Ron.
Kathleen Walch: Hi. We’re so excited to be on your podcast today.
Ron Schmelzer: Hey all, it’s Ron Schmelzer. Good to hear from you and be on your podcast as well.
Bill Raymond: Yeah, really looking forward to this. We’re actually doing a little podcast swap because we both have fairly similar topics that we talk about in terms of managing work, but from a different perspective.
Strategic Planning and Delivery of AI Projects
Bill Raymond: Today we’re going to talk about strategic planning and delivery of AI projects. Before we get started, I’d love that if maybe you could introduce yourselves, maybe start with you, Kathleen.
Kathleen Walch
Kathleen Walch: Sure. I’m Kathleen Walch. I am host of AI Today podcast and also a managing partner at Cognilytica. We have been running AI Today since 2017, now, actually so many years. And we cover AI Today exactly as the name suggests. We’ve seen other podcasts out there that sometimes talk about, you know, AI in the future, AI is sci-fi, or maybe cover hot topics and news.
Kathleen Walch: And we really wanted to have practical applications and share that because there’s a lot that’s going on and some of it is mundane and doesn’t necessarily get as much attention as other topics, and we wanted to highlight that because that really is what’s kind of driving this industry and a lot of the applications forward.
Kathleen Walch: Of course, if your listeners are paying attention to AI at all, they’ll know ChatGPT, and that’s really hot right now, making some people a little nervous, especially schools and academic settings. But there’s a lot of other things that AI is doing as well, and that’s what we cover.
Kathleen Walch: And then a little bit about my background. I started out actually in marketing. I worked for Heart Hanks and we did a lot with Bed, Bath & Beyond actually. So that was when I was introduced to Data, Big Data, the messiness of data. From there, my husband and I had started a startup actually back in 2012, and that was when I met Ron first. And then around 2014 started working with Ron at Tech Breakfast, which was a morning demo series that we highlighted all different sorts of tech. A lot of it was very entrepreneurial, a lot of startups, but some larger companies did present as well some new features, new products that they were creating.
Kathleen Walch: From Tech Breakfast, because we got to see so many different people and companies demo, in around maybe 2016, voice and voice assistance was becoming really hot and really popular. And that’s what got us involved in artificial intelligence to begin with, really kind of moving forward in that direction.
Kathleen Walch: And then in 2017, that was when Cognilytica got started, including our podcast. And we have been covering AI, machine learning, advanced analytics ever since.
Bill Raymond: Well, thank you for that and we’re going to talk about Cognilytica in just a moment. Ron, could you share a little bit about yourself?
Ron Schmelzer
I’m Ron Schmelzer. I’m also managing partner and head here at Cognilytica along with Kathleen, host of the AI Today Podcast, which is going in to be into our sixth year fairly soon, over 300 episodes we’ve been doing. So we understand the podcast hustle well, and as Kathleen mentioned, she did a really good job.
We’ve been following AI since the very beginning of the podcast and what we’ve been covering, but AI goes back to the 1950s. So it’s kind of funny how we talk about the current topics and the current trends, but for those who have been in the AI industry that are like, been there, seen that.
Ron Schmelzer: Of course, things get better and algorithms get better, and systems get better and things get crazier. But we go through these waves and so one of the biggest things that we do on AI Today and in all the stuff that we cover and Cognilytica and all the other things that we do is really focusing on the here and now.
Because there’s always a lot of talk about the future and the possibilities and what can happen, and of course research, which can get a little honestly, complicated and very hard to do that on a podcast. Although there are podcasts that do that, that focus on some of those low level details. I wonder how they do that.
And also really there’s a lot of other podcasts that maybe talk about, some are like the trends and ethical stuff, we do cover that. But if we can’t make AI do anything valuable for us today, then it’ll just go through those waves again and well, we’ll be back here in about 10 or 15 years because that’s the frequency at which AI sort of comes up and then goes away.
Cognilytica
Bill Raymond: Yeah, and we’re going to be talking about strategic planning and delivery of AI projects. I know that Cognilytica focuses on that, so could you just provide a broad brush overview of who Cognilytica is and what you offer?
Kathleen Walch: Sure. So Cognilytica, as we said, we’ve been around since 2017. We’re really focused on AI, educating the market. We have a number of different courses that we offer. One of our main courses is the CPMAI, The Cognitive Project Management for AI Certification. It’s the best practices on how to do AI right.
Kathleen Walch: And many different organizations, government agencies, large corporations, small-medium size organizations have gone through it because they want to know a step-by-step approach for how to run and manage AI projects.
Kathleen Walch: From that, as we’ve talked to different people that have taken it and different customers and clients that we’ve had, we do offer some more fundamental education, some more specific education, like Big Data fundamentals, for example.
Kathleen Walch: We also have an ethical and responsible AI course that we offer, because that’s become quite a hot topic and people want to make sure that they’re thinking about building ethical and responsible AI tools, especially as it’s getting adopted more mainstream and put into practice. So that is, you know, in a nutshell what we offer at Cognilytica.
Kathleen Walch: In addition, we also run a number of different events. Because we wanted to not only talk about it and then educate people, but then show them how it’s actually being applied in the real world. So we haveenterprise-focused events where it’s enterprise and data focused, talking about how they’re implementing Big Data, AI, advanced analytics, and then we have a government-focused event series as well, where we have state and local, federal, and then international governments as well talk about how they are adopting AI.
I guess we should point out right away is that when we say AI we mean artificial intelligence and that could cover a lot of grounds. But I think it would be cool if you could provide some sort of a broad brush overview of what you mean by AI.
AI overview
Ron Schmelzer: That’s a really good place to start and certainly it’s not a bad question, because that is a lot of what we do at Cognilytica in our AI Today Podcast. As a matter of fact, we’re in the midst of a glossary series where we’re defining all of the terms in one little term at a time.
Ron Schmelzer: So we start by talking about the goal of artificial intelligence is to have machines do some of the tasks and do some of the things that otherwise people would be doing because it requires some aspect of cognitive ability, right?
Ron Schmelzer: And of course, there’s different aspects. They generally group into these three areas of perception, which is the being able to understand the world around you, things like recognition and things like that, understanding speech and things like that.
Ron Schmelzer: And then there is prediction, which is the ability to make decisions on things and decide what the next thing there is to do. And planning, which is the ability to look a couple steps ahead. And many of the things that we try to do as humans are difficult for computers when you program them, which is the alternative because you’re telling computers what to do, and ideally it would be great if machines can learn how to do many of these things and a lot of the goals of artificial intelligence have been providing these techniques, these algorithmic approaches combined with data and combined with computing to give machines this cognitive ability to do many things.
Ron Schmelzer: And as we start doing them, they’re like, oh, that’s really cool. Machines can take a look at a picture and understand what’s in it. That’s the pattern of computer vision or recognition pattern. And the reason why I say a pattern is because a lot of the things that we want AI to do, when we say AI, a lot of times we’re talking about different applications of AI.
And so I’m trying really hard not to do the whole lesson here on your podcast, but long story short, there are seven patterns of AI that tend to group the things that we want to do with AI into these like buckets.
AI patterns
Ron Schmelzer: And start at a very high level, there’s the recognition pattern, which is getting machines to understand the world around them, images and speech and things like that.
Ron Schmelzer: There’s the conversational pattern. Which is allowing machines to interact with humans in our natural language.
Ron Schmelzer: Then there’s the predictive and analytics and decision support where you have machines trying to figure out where things are going.
Ron Schmelzer: You have the pattern and anomaly pattern which is machines trying to figure out which things are like each other and which things are perhaps not.
Ron Schmelzer: You have the autonomous systems pattern, you may be familiar with that, which is machines that are able to operate with little or no human control.
Ron Schmelzer: We have the hyper-personalization pattern, which is machines trying to identify individual people on their profiles rather than doing bucketing.
Ron Schmelzer: And then finally, we have this games scenario and simulation goal-driven systems pattern, which is machines trying to figure out the optimal path or solution to a problem.
Ron Schmelzer: And generally all the things we try to do with AI currently are in one or more of those buckets.
Ron Schmelzer: Final thing I’d like to say about that is that the alternative to doing AI for these little things called Narrow AI is this idea of General AI, artificial general intelligence, which is the one system that can understand how to do anything and who can accomplish any task.
Ron Schmelzer: That’s what our brains can do. We have not solved that problem. We have not built the generally intelligent system, although people are trying. So everything we’re doing right now are one of these narrow buckets.
Ron Schmelzer: I know I covered a lot there, but I don’t know if Kathleen, if there’s anything that fell through the gaps, buthopefully that helped.
Seven patterns of AI
Kathleen Walch: Yeah, that was a great overview and we always like to talk about AI in the seven patterns of AI because you’re right, it’s a very broad brush and when I’m talking about AI, I may be talking about autonomous vehicles. You may be talking about a predictive maintenance system. Ron may be talking about computer vision.
Kathleen Walch: All of them fall into that umbrella of AI, but they are really different in their applications, maybe in the data that you need, the algorithms that you’re going to select for it. So that’s why we like to talk about it in the seven patterns.
Kathleen Walch: It also helps you break down your projects a lot better so that you’re really understanding what it is you’re trying to do.
Kathleen Walch: Because we always say, you know, with the CPMAI methodology, start with business understanding, you want to make sure you’re solving a real world problem. And it seems so simple, yet so many people, especially when it comes to AI, somehow forget that. They’re like, this is so exciting, this is so futuristic, we’re just going to move forward. And we’re like, but what problem are you trying to solve? They’re like, we don’t care, it’s so cool. We’re like OK.
Kathleen Walch: So that’s why we always say, think about it from the patterns perspective, think about it from the problem that you’re trying to solve, and then move forward from there.
Bill Raymond: Yeah, and it is always interesting, because obviously we love to work on the cool things first, right? There’s a new thing, it’s fresh and shiny, and you want to try it out. I think that’s a natural human thing to do, but sometimes we have what we need already, or we need to think a little bit harder about it before we jump into it.
AI case studies
Bill Raymond: So if we could kind of pull that together a little bit, you know, our audience are of coursebusiness leaders in organizations and they’re probably thinking about how they can use AI in two different ways, perhaps.
Bill Raymond: One, to improve a customer experience or for their products to add additional value or the other is internal, where they’re thinking about using AI to help with their operations of their company and to make improvements there. Could you provide an example, a case study of the latter, perhaps?
Kathleen Walch: Sure. So, we’ve seen it used with IT Help Desks. We have a ton of examples, so I can share this one, Ron can share another one if he’d like, where you know, you get a lot of calls, you have to figure out what the call volume is to your Help Desk and how many people are calling for simple things like password resets.
Kathleen Walch: And maybe how many people aren’t able to work after hours, because let’s just say the Help Desk works nine to five and somebody’s logged in at 9:00 PM after hours, and they’ve locked themselves out for some reason, which I think we’ve all been accused of doing. And so now they’re like, well, I can’t work and I have to meet this deadline. How can you automate that so that you can have password resets automatically?
Kathleen Walch: That’s what you can do with the help of AI. Use natural language processing, you’re able to have a bot help the person on the other end, and then that also frees up the Help Desk to handle these more complicated and complex situations where they really do need to be involved.
Bill Raymond: Yeah, and Ron, I’d love to hear your thoughts, but just a quick little thought on that, Kathleen. I actually, so I’m a consultant, I help other organizations do work. And this is something that has been a challenge for me for years because sometimes the organization just says, Oh, your password needs to be reset because you’ve hit some three month period or something like that.
And anytime I try to do it, all of a sudden they’re telling me to go to this website in their corporate network that I can’t access because my password’s not working. And I just wanted to share an example of this. One of my clients set up a phone system where you can have a natural language conversation with it, and then it helps you do the password reset outside of the network.
Bill Raymond: It’s still all very secure and all that good stuff, but it just walks you through it. And I thought, wow, that’s such a great use of it. And all I did was say, I’m an external contractor, I need to reset my password. It understood that and it brought me through the process that I maybe wouldn’t be able to see on their internal corporate site.
Ron Schmelzer: I think in general, those are the most useful approaches or applications of AI. The ones where these intelligence systems are these more cognitive ability systems are helping people. This is what we call the augmented intelligence pattern, where you’re not having it go off and do something entirely on its own. One, because these systems aren’t entirely reliable.
Probabilistic. Introducing risk
These artificial intelligence systems are what’s called probabilistic, which means that they may be great 90% of the time, 95%, but they’re not a hundred percent. So whenever you require 100% functionality, like getting into a car and not being in the driver’s seat, being in the backseat and telling the car to drive, that’s when you’re getting into risky territory.
Ron Schmelzer: Or saying, hey computer, generate for me 3000 words of text. I’m going to go ahead and just paste it somewhere. Now, 95% may be okay, but that 5% may not be, and that’s when things get risky.
Ron Schmelzer: So, in organizations you don’t want to be introducing risk, you want to be reducing risk, you want to be improving efficiency, you want to be reducing cost, you want to be improving satisfaction, right?
Ron Schmelzer: These are the measures of success for an organization. And when we’re applying AI, a lot of it comes down to improving things that can be increasingly better automated, even though automation is not the same thing as intelligence, because you can program something to do something repeatedly and that’s automation.
But we can make the automation more intelligent so they’re kind of cutting across each other, right? We can add one to the other. And when we do that, we can do things like have systems that automatically look in our email inboxes and extract things like invoices or expense reports and automatically submit them.
Ron Schmelzer: Or it could be doing things like helping radiologists do their job better, by looking at images, although that has not been proven to necessarily be as successful as we want it to be. Or it could be doing things that’s not driving your car, but making you safer while you drive your car, keeping you in your lane, making sure that you’re breaking in time, things like that.
Ron Schmelzer: So, these are where it’s less sexy because we would love to have some autonomous robot doing all these things, that’s what’s in the movies. You don’t see in the year 2300 an automated email invoicing system that just doesn’t capture anybody’s science fiction interest. It’s boring, right? But that’s where the practicality is.
Ron Schmelzer: And so when we talk about a lot of the AI applications, whether it’s doing things for city governments, where they don’t have a lot of employees and they need to handle needs perhaps at three o’clock in the morning when someone has a real issue, wouldn’t it be nice if there was some sort of automated chatbot system that can handle their request and might not fix it, but it could log the request and get something out in the next morning? That’s appreciated. And I think the interesting thing is those things are going to become more and more part of our expected daily lives. We’ve had this situation where we have a little chat box on our site, which is really meant for somebody to interact with one of us.
Ron Schmelzer: And increasingly, people are thinking somehow that it’s some sort of automated chatbot. I think they’re getting trained to expect an instant response from an automated system, even though there’s a person behind it.
Ron Schmelzer: So, long story short, there’s many, many corporate applications, business applications, practical applications of AI that have to do with this sort of augmented role of machines just helping people do their jobs better and be more efficient.
Ron Schmelzer: We like to say, take the work out of the job and not take the job away so that people are doing what they’re supposed to do in their job and not spending six hours out of every eight hours triaging email or entering something into our CRM or ERP system.
Bill Raymond: Yeah, thank you for that. I really appreciate those examples. I’m thinking about all of the things that you both shared with me, and chatbots I think are fairly well known. We can go on to almost any website now and there’s little chat thing somewhere on the website that says type in your query and we’ll respond.
Bill Raymond: And sometimes they’re really cool and amazing, and sometimes you could tell someone just uploaded a whole bunch of frequently asked questions and it comes out with some basic response and those kind of go across the gamut. But the promise is there, right? That AI is going to help you automate things, help you remove that need to have so many human beings touching something that can easily be automated.
Bill Raymond: But I guess the question that I would have is, everything that you just described about AI and what it has to offer, and now we’re seeing the openness of some of these systems right now, like Open AI’s chat system, ChatGPT or DALL-E, where you can just type in a prompt and get a picture.
Use AI as a tool from your toolbox
Bill Raymond: Now people are starting to think about this and saying, whoa, this could be game changing to my business. And what that can do is send you into a spiral of all the cool possible things that you could be doing. So I wonder, as a leader in your organization, how do you think about these ideas? How do you reflect on how AI might be able to help you and reflect on the ramifications of what you’re going into?
We always like to say that AI is a tool in your toolbox, right? So it’s not going to be the one solution that solves everything for you. Use it as any other tool, understands its limitations, understand what it’s good at, and then make decisions from there because everything has a cost.
As Ron mentioned, you can type something in ChatGPT, say, type me this 3000 word article on project management, and it will give you something that’s pretty good, but it’s not going to be a hundred percent. You still should have a human in there editing it. You still should have a human in there doing something.
Kathleen Walch: So use AI as a tool, and yes, this is what happens, and Ron had mentioned to it earlier where artificial intelligence, I mean, the term was coined in 1956, this is not new. This has been around for decades. And you may say, yeah, but now it’s different, why am I hearing about it now? And it’s the third wave of AI.
Kathleen Walch: So AI has gone through two previous winters, which is a period of decline in investment, in popularity, in funding. And a main reason that it has gone into this hibernation winter period is that we overpromise and then under-deliver on what we can do. So we get really excited, we talk about all the possibilities, we say, oh my gosh, look what it can do. It can, it can write novels, it can write songs, it can write code, it can do all this stuff. And then when you actually put it into practice, you see some of the limitations with it and organizations go, maybe they put something out and then they get in trouble for plagiarism or they get in trouble, which it’s not supposed to be, but you know, it’s not perfect.
ChatGPT is pulling from different sources. We’ve seen articles that some people are getting in trouble for that if they’re not going in and editing it as a human. Also, maybe they’re not disclosing that it was written by an artificial intelligence system. So there’s all these things that you really need to be paying attention to, and we say, don’t let this replace humans. Don’t let this replace thinking. Don’t let this replace your common sense. Use it as a tool.
Unique roles in a team working on an AI project
When we talk on this podcast, on the Agile in Action Podcast, we very frequently will talk about what teams look like, the makeup of a team. And we’ve talked about organizations bringing in agility in human resources, in finance, in manufacturing, and in software development, of course. And there’s a certain type of role that you would see in those different types of scenarios. are there any unique roles that you would see in a team that’s working on an AI project?
Ron Schmelzer: Absolutely. As a matter of fact, that’s actually one of the places that a lot of organizations get tripped up. AI projects are actually not like typical application development projects. If you think about building, say, a website or a mobile app or any sort of something that requires, say, focused on the building part, because it turns out that part is fairly trivial.
You can go and download any sort of chatbot software and we’ll do that. So the question is what makes an AI system work? Well, it’s not the software, it’s the data, right? And the problem is that most organizations haven’t sufficiently invested in roles that focus on data cleansing, data quality, data integration, data annotation, data labeling.
Ron Schmelzer: Without those, the AI systems just simply do not work. Because the old phrase "garbage in, is garbage out" is incredibly true with AI systems. And if you give a system the wrong data to train on, you will basically get the wrong results. Similarly, if you have a lot of great data, you can get a lot of great results.
Ron Schmelzer: I put this out there, what exactly is ChatGPT doing that we didn’t have before? Is it some new algorithm? No. They’re using transformer models, which have been around for a while. What is it? Well, when you look at it, it’s because they have 175 billion parameters. Is this huge network and it trained it literally on the whole corpus of everything they can get on the internet.
Ron Schmelzer: And this is when you know how it works, well it’s like a magic trick. It sort of takes the magic away and you’re like, oh, it’s just a big predictive, you know, when you type on your phone and you type the word first, and then it comes up with what it thinks the next word will be after that. Well, it’s a very, very, very simple version of the same thing.
Ron Schmelzer: When you give ChatGPT a prompt, it’ll come up with the next thousand words of what it thinks it’s next. Now, the thing is, it’s not just creating these words out of scratch. What it’s doing is it’s almost like we call it like a very intelligent parrot. It’s just going back and be like, oh, I remember Pollyana.
Ron Schmelzer: Next thing is cracker. So Polly goes cracker, but it goes continues, right? Or you could think of it as like it’s going to assemble a collage of all of this data, this training data that’s had before, and it’s going to assemble this collage by sniping things out of this magazine and that magazine, and next thing you know, you got 3000 words.
Ron Schmelzer: Now why go into all this is because it’s the data. And the roles that are typically required, the most important roles in an AI organization are data engineering, data science, data quality and data quality management, data governance.
Ron Schmelzer: And you might think, well, these are things, I just need a machine learning engineer, I need a developer. Those are the least important roles. I can go and download a Python toolkit in five seconds that no developer in your corporation will even be able to improve on. So what’s the point? What you can’t download from sci-kit or from any of these places is the data cleaning, the data collection, the data annotation.
Ron Schmelzer: So the most important roles, like the fundamental key roles are data roles. And this is also why in a methodology and an approach to running an AI project has to be data centric. And this is where even the smartest people in AI are getting tripped up.
Ron Schmelzer: They’re saying "Why are my autonomous vehicle systems not working? Why is my image recognition system for my medical x-ray thing not working?" Said Andrew Wing, the guy who is the deep learning person, very well known who head of AI for Baidu and Google and it’s created Coursera’s Deep Learning course. Why doesn’t my X-ray system work? And then he’ll tell you, it’s because of the data.
Ron Schmelzer: So how did they get that far without doing it? So most organizations don’t have the millions of dollars that Google and Microsoft and Facebook and all, and Amazon, those companies have. They don’t have the 10,000 employees that Amazon had in their Alexa division to solve these problems. You usually only have a handful of people, so you don’t have that many chances and that many resources to get it wrong.
Ron Schmelzer: So get it right. Focus on the data and we tell people learn how to do it right. But you know, you know how it is, people like to roll ahead with implementing before they think about the harder stuff.
I think about the term that you’ll hear software developers use all the time, which is technical debt. Sometimes you build this software product, and it’s been around for so long because it’s become ubiquitous. It has a lot of old code in it that it prevents you from innovating.
Bill Raymond: And so you almost have to start taking apart that old code and fixing it and reworking it so that you can do other things with it. And then famously Microsoft Office was on the desktop when all of a sudden everyone said, well, we want to do our word processing online. You can’t just rip up all of Microsoft Word and say, now it’s on the browser.
And I think there’s a lot of work, a lot of data science that went into it. A lot of technical capabilities had to be invented in order to do things like that. And I look at what you are saying as we have another thing that’s similar to technical debt, which is maybe that we did not design our data systems the way that we need to.
Bill Raymond: We always talk about how I need this system from my piece of the business that manages my finances this way, and by the way, there’s no special way to collect that data with another system that’s related in order for AI to work. And it’s almost like, this is actually going to turn into a really big project.
Bill Raymond: Now we’re talking about spending millions of dollars to make the data similar or to purchase a new system and to have to tie the data together so that the AI can work on it.
Bill Raymond: I can imagine that this kind of a simple idea of working with some company data to do something with AI could blossom from, a very, let’s just test this out to a very large, long, drawn out effort.
Tehnical and data debt
Ron Schmelzer: Absolutely. There’s actually a well known problem that builds off of technical debt called data debt, right? And the idea with data debt is, it’s actually far worse of a problem than technical debt. With technical debt, you may be like, well, I’ve already invested in all this code, I have all these systems. So every single time you want to make a new update, you’re like, I have to go back and fix everything.
Ron Schmelzer: And of course, the technical debt problem does get worse and worse over time. And then you’re so tempted just to throw out the old, just get rid of it and rebuild a new one. And then all of course you do is you just create more technical debt.
Ron Schmelzer: The data, that problem is actually worse. Because what happens with data is that we don’t like to get rid of it, so we end up saving it and storing it in all these different systems. And we also have them in different formats.
Ron Schmelzer: And so what ends up happening, the data debt problem actually grows exponentially, because not only do I have to deal with the fact that I have lots of data in lots of different places and lots of different formats, I have to secure it. I have to back it up. I have to prevent it from getting stolen. So it turns out that the data debt problem is significantly and probably even exponentially more difficult unless you have some sort of approach for managing data, which of course most organizations don’t.
Ron Schmelzer: What ends up happening is that you get these interesting cultural or you get these fights within organizations where people try not to share data because they’re afraid of people copying it and then just making things even worse. Or having security issues or privacy issues or compliance issues. The data debt problem is a very significant problem, but that relates to how you manage a project.
Ron Schmelzer: Because what you don’t want to do in a project is basically say, oh, we have this great idea to do this thing, all we need is access to this data, and we’ll do this, and this, you know, this stuff. And then that other person said, hold up, I’m not giving you access to that data. Well, that just killed your whole project, didn’t it?
Ron Schmelzer: So, I know Kathleen, we talk about this all the time in projects, dealing with projects.
Kathleen Walch: We do, and we also talk about project scope, understanding the data, understanding if you have access to that data and really try and narrow the scope of your project. I think that scope creep is real. It can continue to happen. And also sometimes projects, they can go on forever if you don’t have an end that you are saying, okay.
Kathleen Walch: AI projects, they do need to be maintained, you can have data drift, you can have model drift, but you might not need as large of a team on it. So I’m not saying, it’s going to just go away, you build your model, you put it out there and never look at it again. But when you’re building it, you do want to make sure that you are scoping your project correctly.
Kathleen Walch: That’s why it’s so important to follow a best practices methodology. That’s why it’s so important to understand even what it is you’re trying to do. Because if you’re like, I just want to do an AI project. Okay. What’s your main goal? What’s the ROIs that you’re looking to get from this? What data do you have?
Kathleen Walch: You need to be making sure that you’re asking all these questions, otherwise it’s going to get very big, very complex, very overwhelming, very quickly. And then we’ve seen far too many AI projects fail.
Kathleen Walch: In fact, there’s statistics from a bunch of different sources, anywhere from 70 to 85% of AI projects ultimately end up failing.
Kathleen Walch: And it shouldn’t be that way, but it’s because they don’t go in with actually solving what is the business problem that I’m looking to solve? What’s the ROI that I’m expecting to get? Is that going to be higher than the cost of my project? Do I have the data that I need? What does it mean to make sure that I have data in a state that I need in order for me to have these systems learn? Because we want to make sure you’re learning on that good quality data.
Kathleen Walch: So a lot of people are missing those steps, those very important questions and critical factors they need to be asking, and that’s why we’re seeing these projects fail.
Bill Raymond: Yeah, and I can certainly understand why. And we are so early days in terms of, even though it might be around, it might have been around since 1956, I think we’re in early days with people generally that aren’t in the AI space, getting to understand what AI is. And so maybe more eyes are open, but we’re still excited and we’re not quite sure how to properly manage an effort like that.
CPMAI or Cognitive Project Management for AI.
This leads us into the conversation around CPMAI or Cognitive Project Management for AI.
Bill Raymond: This is something that you offer at Cognilytica and I think it tries to address some of the problems you just laid out. Can you describe CPMAI a little bit more?
Ron Schmelzer: Yeah. And I think a good way of understanding it, and it is a lot of letters and we apologize for those folks who have heard this for the first time, trying to memorize it.
Ron Schmelzer: But really, the idea is it’s project management for AI. So those are at least the four letter parts. And the C is cognitive, maybe originally it was Cognilytica, but let’s just say it’s project management for AI.
The main idea here is that it actually builds upon an approach, a framework, a I don’t want to call it a methodology because actually it’s just a process for running data mining projects that was around in 1999 started something called CRISPDM, which is the Cross Industry Standard Process for Data Mining. The idea with CRISPDM was that back in the day, in the late nineties when you were trying to do any kind of analytics project on a Big Data system, back when Big Data systems were slow and cumbersome, and perhaps the people who needed to interact with them were highly specialized that you needed to be able to formulate the data query or the data project in advance, so that way if it took two weeks to get the response, you weren’t burning months and months. So they came up with this approach to running these data-centric analytics projects.
But the problem was, is that they didn’t update it since the late 1990s. And here we are in the 2000s when basically we’re like, wait a second, we need to run AI projects.
Ron Schmelzer: And amazingly, they’re just a fancy form of Big Data project, a very specific form. In the mid 2010s, we and others basicallyevolved the CRISPDM approach to become CPMAI, which is the Cognitive Project Manager for AI, which builds upon the same idea of these six iterative phases, that’s what they’re called in CRISPDM. We understand the word phase may be an issue for some, but it’s these six steps that must be followed in a project, but they’re iterative in that they can be, you can go back and forth when you realize you don’t have enough data for something, or the method that you selected for doing analytics might not be appropriate.
Ron Schmelzer: You’re allowed to go back. You don’t have to push out your project with the bad data or bad algorithm. We can go into that now, but basically what CPMAI is, is this prescriptive approach that’s iterative for running AI projects that’s data centric and agile.
Ron Schmelzer: For your listeners, iterative would probably be the best way to think about it.
Iterative approach
Kathleen Walch: And it’s important to think about it that way because when we talk to organizations, they’re telling us that it’s taking 9, 12, 18 months to go from start to finish just on one iteration. And we’re saying, that is not an iterative approach. That sounds a little more like waterfall to us and that’s not how you should be running these projects.
Kathleen Walch: So it starts off with business understanding. Figure out the problem you’re actually trying to solve. Then data understanding. So we’re going to think about our data environment, our data sources, data quality issues. Then we have data preparation. So this is about actually preparing our data, labeling it if we need.
Kathleen Walch: Then we have data modeling, so this is about algorithm selection, training and tuning our model. Then we have model evaluation. So once we’ve built it, we want to make sure that it’s performing the way that we think that it’s going to be performing. And then we have model operationalization. So this is putting the model into the real world.
Kathleen Walch: How are we going to actually be using it. Those are the six phases.
Kathleen Walch: As we mentioned it is iterative, and it’s supposed to be a step-by-step approach. When we talked earlier about scope, start small. You know, if you’re building a chatbot, for example, an AI-enabled chatbot, what is the number one question that is asked of your chatbot and have it address that first.
Think big, start small and iterate often
Kathleen Walch: It doesn’t need to be able to answer 10,000 questions, start with one. And then continue in each iteration to bring in an additional question, maybe a few more questions. And that’s how we always say, think big, start small and iterate often. So you’re thinking big because you want to build this chat bot, but you’re starting small with just answering one question, and then we’ll get more and more, and then you’re continuing to iterate so that over time this chat bot is improving and learning, and able to answer more questions.
Kathleen Walch: When you do it that way, you’re going to actually see results pretty immediately and you’re not going to be having these projects that take 12 months to get that first question answered, because a lot of things change in 12 months as we’ve known from living these past few years with the pandemic.
Kathleen Walch: And also maybe teams are changing, people are leaving, so you really want to get it out there. Also, upper management, depending on how much money you’re spending on this thing, they may say, we’re not seeing results, we’re canning the project. And then all of that work and effort that you’ve done goes to waste.
Bill Raymond: Well, and I have to imagine that by starting small with, I liked your chatbot example where you start answering one or a few questions and then you can move on. The team members, the people involved in those projects may have never worked on an AI project. So that’s good learning for them, where they can see where the data that they’re providing is good, solid, usable, workable data, or if they really need to think more about how they’re going to present it. And it gives everyone a comfort level that they’re starting to understand what some of the limitations are, what some of the capabilities are, and then they can start thinking about, okay, this is how we need to feed the system.
Bill Raymond: Because that very often, that’s what gets lost, right? We don’t know how the tool works and we have to learn that. And some of us can learn just by reading a book and we’re good to go.
Bill Raymond: Others we have to learn by doing and of course with anything, if it’s a new technology, there’s going to be a learning curve anyway. And so I love this whole concept of starting small and growing, using these small iterative approaches. Because it’s not just to show value to your senior management, it’s also Now they can share their experience and the next team that takes us on can learn from that experience as well.
Learning the method
Ron Schmelzer: Yeah, and it’s not just learning the technology and learning the ropes of that. It really is also learning the right way to do things. I don’t know why that’s such a struggle. People will invest hundreds of thousands of dollars in tools and tens and hundreds of thousands of dollars in data and data preparation, but they won’t spend like a penny on method, learning the right way. They’re like, I’ll just figure it out as I go along.
Ron Schmelzer: It’s embarrassing the number of C levels and heads of data departments where we say, what approach is? How are you using to run your AI projects? And they’re saying, we don’t use any standard approach, we’re using some ridiculous approach, which doesn’t even bear repeating, you know, learn the method!
Don’t you want to know what Google figured out? Don’t you want to know what Intel ended up doing? Don’t you want to know what these major firms learn? And they go, don’t make our mistakes. But I hear people are like, nah, I’d prefer to make a whole bunch of mistakes. And I’m like, guys, education. I know we have something to sell, we’re selling education, but we’d only be selling it if people were using it, were needing it, right?
Ron Schmelzer: And it’s the same thing, you go to school, you go to school to learn math. You don’t be like, I’m going to figure out math as I go along.
We can go into any C level right now, any Fortune 1000 organization and be like, there’s a small percentage of you that are doing it right and a very large percentage of you that are not.
Ron Schmelzer: And yeah, we tell people, invest, invest in education, invest in training, and get a few people certified. Do it the right way. You know, there’s no argument for not doing it the right way.
Bill Raymond: We were just talking at the beginning of this podcast that we use AI to edit our podcasts. I don’t tell people I use AI to edit my podcast unless I’m talking to AI people like yourselves. What I say is, I have this great tool that recognizes the voices and automatically labels them and allows me to edit the podcast. This is of a document instead of trying to understand audio and video systems. And all these capabilities are AI based, but really what we’re seeing is that they’re useful to our organization. And if anyone thinks that something like ChatGPT is going to take over the world, I’m going to share a AI generated joke with you. You ready?
Ron Schmelzer: Sure I’m ready for it. Listeners, are you ready for it?
Bill Raymond: Why did the AI project fail? Because it had too many bugs and not enough debugging. Right? Uhhuh? So don’t worry comedians, ChatGPT is not going to take over your job.
Was that, you basically asked ChatGPT to write the joke?
Bill Raymond: Yes, I did.
Ron Schmelzer: Yeah, see that’s why.
Ron Schmelzer: As a matter of fact, we actually had an interview in one of our podcasts that said that everybody’s like worried about the super intelligence and machines taking over and they actually said, you should only be worried when AI learns to tell a good joke.
Ron Schmelzer: So what you just said was, I think nobody laughed, I think you should all feel comfort because that means AI ain’t be takin’ over anytime soon, so there you go.
Bill Raymond: However, I am going to slip in, that one of the questions I asked you was AI generated.
Kathleen Walch: Oh wow.
Bill Raymond: And that question is " Who are the key stakeholders and what are their roles in a new AI effort?"
Ron Schmelzer: That was a good question.
Bill Raymond: And so when I asked that earlier, probably not exactly those words, that was from an AI generated question.
Ron Schmelzer: Cool.
Bill Raymond: As we’re wrapping up, I’m going to ask you if it’s okay to reach out to you and how people can contact you and learn more.
Bill Raymond: But I thought I’d do one more fun little activity with you, if you don’t mind. Now we are on a podcast, I fully understand that, and this video may or may not see the light of day.
Bill Raymond: However, I am going to show you a video and it’s going to play some audio. We’ll see how it comes out. If it doesn’t come out good, then I’ll put the audio right into the podcast for you. I’m going to play this and I’m going to ask you if you could figure out what elements are AI generated and which elements are not.
Bill Raymond: You ready?
Kathleen Walch: Okay.
Bill Raymond: All right.
Ron Schmelzer: You put us on the spot.
Bill Raymond: And this isn’t a test.
Kathleen Walch: I know.
Bill Raymond: This is actually a fun little thing. Don’t worry, this isn’t a test.
Ron Schmelzer: It is getting harder and harder to tell.
AI Generated Content: I know. All right, so here we go. In a world where technology advances at lightning speed, two powerful podcasts join forces to bring you the ultimate guide to success. From the Agile in Action with Bill Raymond Podcast learn how to improve the value your team delivers to customers using cutting edge agile techniques. And from the AI Today Podcast discover the secrets to delivering artificial intelligence projects on time and on budget.
AI Generated Content: Together the Agile in Action and AI Today Podcast will take you on a journey to the forefront of innovation and help you stay ahead of the competition. Don’t miss this dynamic duo available now on your favorite podcast app.
Ron Schmelzer: Yeah, that whole thing was generated. Yeah. I’m thinking Lumen five. I got a little bit of the, yeah, maybe, I don’t know how much the script you might have written on your own. Maybe. Maybe…
Kathleen Walch: I think you probably prompted it with obviously the titles that we are podcasts.
Maybe there’s some element of you gen, but the rest of it was all generated. Because it’s like yeah, for those of you that can’t watch the video, there’s lot of random stock art and there was a lot of in the future where…
Bill Raymond: Yeah,
Ron Schmelzer: "In the world" but it’s getting better, it’s getting better.
Bill Raymond: Yeah. Yeah. The video is actually just me popping some video in. The script itself was 100% written by ChatGPT, and the voice of course, was a voiceover AI.
Bill Raymond: But, you know, if I played that for you and you didn’t necessarily know that it was AI written, I don’t know that you would have thought that I didn’t maybe have someone read that for me.
Let me tell you, I mean, this is a thing, a lot of the ads, especially the pharmaceutical ads, which are, I tried to tell you some of the worst advertising out there. I don’t understand how, it’s like ask your doctor about Pros Laxa. It’ll… you know, those can be 100% AI generated and nobody will know the difference because the stock art is just like random people walking in a field, and it’s just like the voice is a generic voice.
Ron Schmelzer: I think those will be the first ads you will see on television.
Kathleen Walch: If not already.
And things like that movie, same thing, even movie trailers. We did interview someone who said that a lot of the movie trailers now are being AI generated, scripted and they’re using real footage from the movies, but like it’s selecting the order and doing all that sort of stuff.
Kathleen Walch: So I think it was an interview on the AI Today podcast with the Chief Technology or Chief Data Officer of 21st Century. Right? Cono, Basse.
Ron Schmelzer: Yes, that’s it.
Ron Schmelzer: He was talking about how they’re already doing all that. But you know what, it will be available, I’m not trying to plug any products here, but we’re big users of a product called Canva, which is a really nice image and other generation tool. We would not be able to produce anything nearly as good.
Ron Schmelzer: And they’re adding all sorts of AI elements into it now. It just makes stuff look so good, I mean, I think you’d be foolish now to use that. And I think that’s where things are. We both have kids, Kathleen and I in elementary school and middle school. and the teachers now are like totally onto kids generating stuff.
Ron Schmelzer: So I think the biggest impact is going to be in schooling. I think the days of the essay are over.
Kathleen Walch: They’re talking about writing them in person. Ron has a middle schooler and I think that’s affecting him a lot more than my kindergartener, where we’re still writing things by hand, but give it a few more years, right? They’re going to become a lot more computer savvy. Maybe be writing some reports on the computer and will it all be their writing?
Ron Schmelzer: This is all the stuff we talk about on the AI Today Podcast, so…
Bill Raymond: Yeah, and we’ll definitely provide a link to the AI Today Podcast on the Agile in Action website and of course on the podcast app that you might be listening to right now. And also is it possible for others to reach out to you, Kathleen and Ron directly?
Kathleen Walch: Yes, absolutely. You can find us both on LinkedIn. Type in Kathleen Walch, or Ron Schmelzer and you can also go to cognilytica.com. So that’s C O G N I L Y T I C A.com and you can reach out to us directly there as well. We’re both very active on LinkedIn, and we do get a lot of people who reach out to us from the podcast, so we’d love to hear from anybody that wants.
Ron Schmelzer: We actually have a direct link, if you want to go to AItoday.live, you’ll get right to the AI Today podcast landing page. We have some cool things we do for our listeners. We have some free courses, we have some other content. We’re in the midst actually, of redoing a lot of that stuff. Reach out to us in any of those ways, send us an email if you want info@AItoday.live and we will get it.
Bill Raymond: Wonderful, thank you. And again, that will also go on the agileinaction.com website. And if you’re in a podcast app right now, just go down to the show notes, the description, and you’ll see all those links there.
Bill Raymond: Kathleen and Ron, this is a wonderful conversation. Thank you so much for your time today.
Kathleen Walch: Thanks so much for having us.
Thank you for listening to the Agile and Action Podcast with Bill Raymond. Subscribe now to stay current on the latest trends in team, organization, and agile techniques. Please take a moment to rate and comment to help us grow our community. This podcast is produced in affiliation with Cambermast LLC, and our executive producer is Reama Dagasan.
If there is a topic you would like Bill to cover, contact him directly at bill.Raymond@agileinaction.com.